Artificial Intelligence and Machine Learning
Building Machines that Understand, Learn, and Reshape the World Alongside Humans.
Table of Contents
Module 1:Neural Networks—The Brain of AI Systems
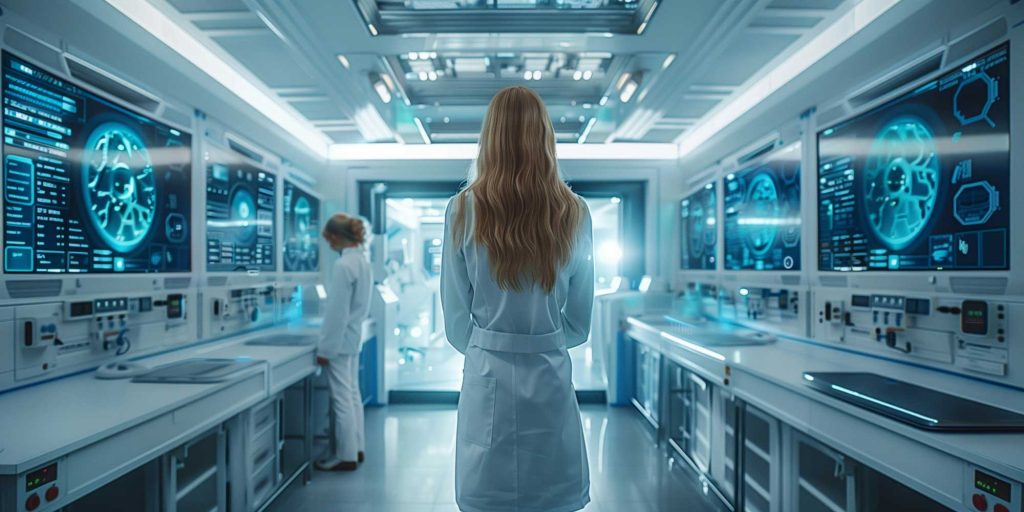
Orion and Nia stood in the center of Synapse Lab, the heart of AI research in the city of Neon Valley. The lab was a marvel of technology—sleek, bright white surfaces reflected the glow of countless holographic screens, each displaying streams of data as humanoid robots moved about with seamless precision. Every task, from managing energy resources to monitoring the city’s infrastructure, was handled by the artificial intelligence systems developed within these walls.
“Okay, Orion,” Nia said, her voice tinged with excitement as her glowing tattoos pulsed with a faint blue light. “Today’s the big day. We’re testing the latest neural network model.”
Orion adjusted his holographic tablet, watching the AI’s neural patterns unfold on the display. “I’m ready. But let’s be honest, Nia, neural networks are still a mystery to most people. It’s like trying to explain the human brain to a computer.”
Nia smiled, her eyes twinkling as she connected her wristband to the main server. “True. But neural networks are like the brain of AI. It’s how these machines think, learn, and make decisions. Let’s show the world what this new model can do.”
Neural Networks: The Brain of AI
As they activated the system, the holographic display projected a massive neural map. It looked almost like a web of light, nodes connecting and glowing as the AI processed information. Each node represented a neuron, and the pathways connecting them were the “synapses,” mimicking the way a human brain functions.
“So, what exactly are we testing today?” Orion asked, his fingers flying across his holographic tablet.
“This new neural network model is designed to think more like humans,” Nia explained. “It’s been trained to recognize complex patterns and make predictions based on data—just like how we learn from experience. The cool part? It can handle tasks we usually think are too abstract for machines.”
Orion nodded. “Let’s start with something simple. We’ll test its ability to recognize objects.” He tapped the screen, and a humanoid robot in the corner of the lab activated.
The robot’s vision system connected to the neural network, and objects started to appear in the air, projected as holograms. A coffee mug, a book, a plant—each object presented to the robot was instantly analyzed and identified by the AI.
“Look at that,” Nia said, her tattoos glowing brighter. “It’s not just recognizing the objects; it’s understanding their context. The AI knows the mug is used for drinking, the book is for reading, and the plant is part of the environment. Neural networks make that possible.”
From Learning to Reasoning
As the test continued, the neural network was pushed further. Now, it wasn’t just identifying objects—it was learning to reason.
“We’re going to introduce a decision-making scenario,” Nia said, pulling up a new interface on her wristband. “Let’s see if the AI can help solve real-world problems.”
The holographic lab environment shifted, and the city’s power grid appeared before them. Neon Valley was known for its energy-efficient design, but an unexpected surge in demand was straining the system. The neural network now had to decide how to allocate resources without causing a blackout.
Orion watched in awe as the AI analyzed the data in real time. “It’s learning the same way we do. By trial and error. But unlike humans, it can process thousands of possibilities in seconds.”
The AI made its decision, rerouting energy in a way that balanced the system and prevented an overload. Nia tapped her wristband again, locking the solution in place.
“That’s neural networks at work,” she said. “It’s like we’ve given the AI a brain that can think, learn, and reason. But the question is—what’s next? How far can we push this technology?”
The Future of Neural Networks
As the test ended, Orion stood by the holographic display, still processing what they had witnessed. “So, this neural network—it’s not just about following commands, is it? It’s about giving AI systems the ability to adapt, to grow, to become more… human.”
Nia’s tattoos pulsed once more, a reflection of her own excitement. “Exactly. Neural networks are just the beginning. The more data we feed them, the more they’ll learn. Eventually, AI won’t just be tools—they’ll be partners, reshaping the world alongside us.”
Orion smiled as he watched the robots continue their tasks seamlessly. “We’re not just building machines. We’re building minds.”
Nia grinned. “And this is only the start. Neural networks are the brain, but we still have to decide how we’ll use that power.”
As they stood in the pristine lab, surrounded by the hum of innovation, both of them knew that they were on the brink of something much bigger than anyone could have imagined. The future of AI wasn’t just about intelligence—it was about understanding.
Module 2: AI Ethics—Navigating Moral Dilemmas with Machines
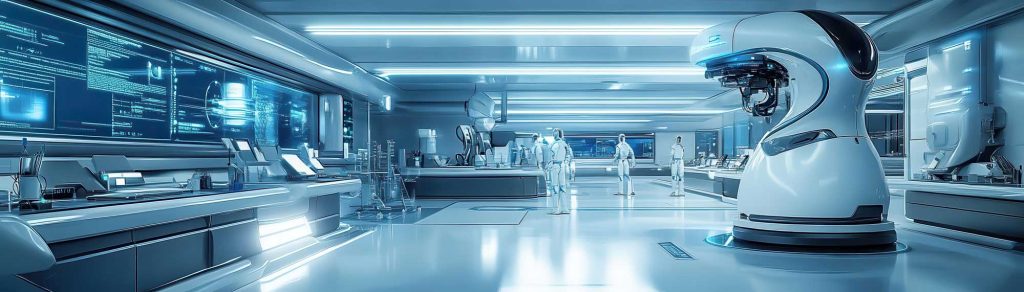
Orion and Nia stood in the heart of Synapse Lab once again, the hum of AI-powered systems creating an ever-present background rhythm. But today was different. On one side of the lab, a humanoid robot stood completely still, awaiting its next command. Unlike the usual technical excitement in the air, a more serious conversation was about to unfold.
Nia’s tattoos glowed with a soft, cool hue as she examined a floating holographic interface. Her gaze was focused, her usual enthusiasm tempered by the weight of the topic.
“We’ve reached a point, Orion,” she began, “where the technology is no longer the issue. The real challenge now is the ethics behind how we use these machines.”
Orion, standing next to her with his holographic tablet, nodded in agreement. “We’ve taught these systems to think, to learn, to solve problems. But what happens when they need to make decisions that involve more than logic? What about decisions that have moral implications?”
The First Ethical Dilemma: The Self-Driving Car
The lights in the lab dimmed as the AI system projected a holographic scene in front of them. A self-driving car was speeding down a busy street, but an unexpected obstacle appeared—pedestrians in a crosswalk and a delivery truck stopped in its lane.
The AI car was programmed to avoid collisions, but in this scenario, it had to make a split-second choice: hit the truck or risk hitting pedestrians.
Orion frowned as the scene played out. “This is one of the classic ethical dilemmas—known as the ‘trolley problem.’ What should the AI prioritize? Minimize harm, or protect certain lives at the expense of others?”
Nia leaned forward, her glowing tattoos flickering as she contemplated the scenario. “It’s not just about logic. This decision involves values—human values. And we can’t expect AI to make the ‘right’ decision without us first defining what ‘right’ even means.”
The AI car displayed its decision: it swerved to avoid the pedestrians, crashing into the truck with minimal damage to both parties.
“This outcome makes sense,” Orion said thoughtfully, “but what if the truck had been carrying dangerous materials? What if the pedestrians were jaywalking? No matter what decision the AI makes, there are layers of moral questions.”
Nia’s voice was calm but serious. “This is why we need to teach AI not only how to think, but how to understand the ethical weight of its decisions. But can machines ever truly understand morality?”
The Second Ethical Dilemma: Surveillance Systems
The next holographic scene shifted to a city plaza where an AI-powered surveillance system was monitoring public spaces for security purposes. The system detected unusual behavior from a group of people and flagged them as potential threats based on their movements and appearance.
Zuri crossed her arms. “Here we go. AI judging people based on patterns—what could possibly go wrong?” she said with heavy sarcasm.
“This is another ethical dilemma,” Nia explained. “Surveillance systems can help prevent crime, but they’re also prone to bias. What happens when the AI makes assumptions based on incomplete or biased data? Are we okay with letting machines decide who is suspicious and who isn’t?”
Orion looked concerned as he adjusted the holographic display. “Surveillance AI could easily discriminate without even realizing it. People could be flagged unfairly based on their clothing, body language, or cultural behaviors. The question is—who’s accountable when an AI makes a mistake? The system, the creators, or the society that lets it happen?”
Nia’s tattoos pulsed as she responded. “That’s exactly the point. The ethical burden doesn’t just fall on the AI itself—it falls on us, the people who build and use it. How do we build fairness and transparency into a system that can potentially infringe on personal freedoms?”
The Third Ethical Dilemma: AI in Healthcare
Next, the hologram displayed a futuristic hospital room where an AI system was responsible for diagnosing patients and recommending treatments. The AI quickly scanned the data of two patients: one, an elderly man with a low chance of recovery, and the other, a young woman with a high chance of survival but in need of an expensive procedure.
The dilemma: The hospital only had the resources to treat one of them. Which patient should the AI prioritize?
Orion rubbed his chin thoughtfully. “This is a tough one. If we leave it to pure logic, the AI might prioritize the younger patient because the chances of survival are higher. But what about the value of the elderly man’s life? Should that be a factor?”
Nia’s voice was soft but clear. “The problem with AI in healthcare is that it doesn’t have empathy. It can make decisions based on data, but it lacks the emotional depth that we humans bring into these situations. Yet, we’re asking machines to make choices that have profound impacts on people’s lives.”
As the AI displayed its decision to prioritize the younger patient, the holographic display paused, awaiting their input.
Orion sighed. “Machines can’t feel the moral weight of a choice like this. But they’re increasingly becoming part of life-or-death decisions. We have to be very careful about where we draw the line between letting AI assist and letting AI decide.”
The Fourth Ethical Dilemma: Autonomous Weaponry
The final scenario appeared—a battlefield where AI-powered drones were deployed. These autonomous drones were given the ability to identify threats and eliminate them without human intervention.
Orion’s face hardened. “Autonomous weapons. This is one of the most dangerous areas of AI. Do we really want machines making decisions about life and death in combat?”
Nia’s tattoos flickered with agitation. “The ethical issue here is control. Once you give machines the power to make decisions about taking lives, you’ve crossed a line. Even if we build in safeguards, who controls the machines? And how do we ensure they don’t make catastrophic mistakes?”
Orion crossed his arms. “The scary part is, it’s not just about what the machines do. It’s about how easily humans might lose their own ethical compass by handing responsibility over to machines.”
The holographic battlefield faded, leaving behind a heavy silence.
Navigating the Future of AI Ethics
Back in the stillness of the lab, Orion and Nia stood, the weight of the ethical dilemmas still heavy in the air. The technology they were building had the power to reshape the world, but at what cost?
Nia’s tattoos dimmed as she spoke quietly. “These aren’t just hypothetical questions anymore, Orion. AI is already being used in self-driving cars, healthcare, surveillance, and even weaponry. And we’re the ones responsible for guiding the ethical use of these machines.”
Orion nodded, his expression somber. “The challenge isn’t just building machines that can learn. It’s ensuring that what they learn, and how they act, aligns with the values we want to uphold as a society.”
Nia’s eyes were filled with determination. “We have to build ethics into the core of AI, not as an afterthought. Because once the machines start making decisions on their own, we won’t be able to control the consequences if we haven’t laid the right foundation.”
As they stood in the futuristic lab, surrounded by the machines they had created, both Orion and Nia knew that the true challenge of AI wasn’t just technical—it was moral. And the future depended on how well they navigated this complex and uncertain ethical landscape.
Module 3: Machine Learning in Healthcare and Medicine
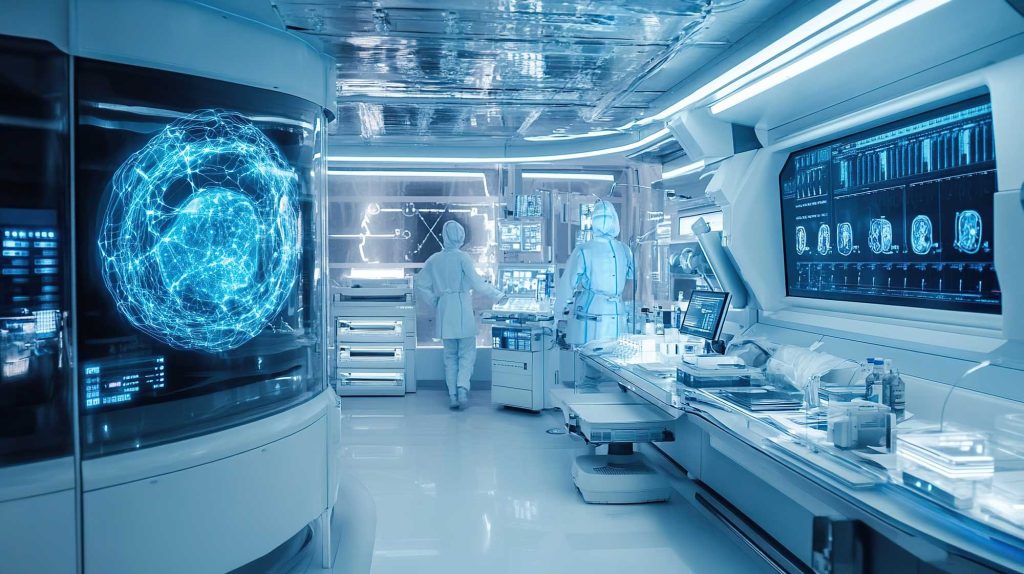
Orion and Nia stood in front of a vast holographic display in the center of MedSys, a futuristic healthcare facility powered by AI. Unlike traditional hospitals, MedSys was a seamless fusion of technology and medicine—robotic arms assisted surgeons, AI predicted medical conditions before symptoms even appeared, and machine learning algorithms helped design personalized treatment plans for patients.
Nia’s circuit-like tattoos glowed in steady pulses as she watched the data flowing across the display. “AI is changing everything about healthcare, Orion. It’s not just about making diagnoses anymore—it’s about predicting and preventing illness before it even starts.”
Orion, holding his holographic tablet, reviewed the latest data on patient outcomes. “It’s incredible how far we’ve come. But I’m still amazed at how machine learning can adapt to each individual. It’s like these systems are learning how to heal in real-time.”
AI-Assisted Diagnosis: A New Era in Medicine
Their first stop in the facility was the diagnostic center. The room was filled with advanced AI-driven diagnostic machines that scanned patients’ medical histories, genetic information, and real-time health data to offer immediate and accurate diagnoses.
Nia gestured to the sleek machine in front of them, a diagnostic AI named Medica. “This AI can process millions of data points from a patient’s history and compare them with billions of global medical cases. But instead of just matching symptoms to conditions, it learns over time, improving its accuracy with each diagnosis.”
They watched as a patient’s data flashed onto the holographic display. Medica scanned the information and presented a diagnosis, suggesting an early stage of a rare condition that might have gone unnoticed for months.
“That’s the power of machine learning,” Nia explained. “It’s not just reactive—it’s predictive. By learning from millions of cases, it can see patterns that even experienced doctors might miss.”
Orion smiled, impressed. “It’s almost like having the collective experience of every doctor in the world in one system. But do patients trust the machine?”
Nia shrugged. “Some do, some don’t. But as the technology proves itself, we’re seeing more people accepting AI as part of their healthcare team. After all, it’s not replacing doctors—it’s empowering them.”
AI in Personalized Medicine: Tailored to You
Next, they entered the Personalized Medicine Lab, where patients’ treatment plans were designed specifically for their unique biology. A large holographic screen showed data streams of patients’ genetic information, lifestyle choices, and health histories.
Nia’s tattoos glowed as she accessed the patient profiles. “This is where machine learning really shines. We’re no longer using generic treatments. Instead, AI analyzes a patient’s genetic makeup and predicts how they’ll respond to different medications.”
Orion tapped on his tablet, pulling up a patient profile. “So, it’s customizing treatment plans? Like, instead of prescribing the same dosage of a drug to everyone, the AI is figuring out the perfect dose based on each person’s body?”
“Exactly,” Nia said, her voice full of enthusiasm. “And it’s not just medication. It’s predicting how lifestyle changes—like diet and exercise—will affect their health. It can even simulate different scenarios, showing how a person’s health might evolve based on their choices.”
Orion watched the data streams shift as the AI calculated optimal treatment plans. “So, machine learning isn’t just reacting to illness—it’s tailoring healthcare to each individual, creating a personalized roadmap for health.”
Nia nodded. “It’s like the AI is learning each person’s health story, and then writing the best possible ending.”
Predictive Medicine: Stopping Illness Before It Starts
As they walked deeper into MedSys, they arrived at the Predictive Medicine Unit, where AI was used to predict future illnesses based on a patient’s current health data. This was the most advanced part of the facility, where machine learning algorithms analyzed real-time data from wearable devices, lifestyle habits, and genetic predispositions to predict diseases long before symptoms appeared.
Orion scanned the data flowing across the holographic interface. “So, we’re predicting illness before it even happens? Like, the AI can tell if someone’s going to develop heart disease or diabetes years in advance?”
Nia’s eyes gleamed with excitement. “Yes! That’s the beauty of predictive medicine. The AI learns from patterns in the data—like blood pressure changes, sleep patterns, or genetic markers—and it can flag potential issues long before they become serious.”
She pulled up a case file of a patient whose real-time data showed subtle signs of stress and hormonal imbalance. The AI predicted that if left unchecked, the patient was at risk of developing a chronic condition in the future. The system recommended lifestyle changes and preventive measures.
Orion marveled at the display. “We’ve always treated medicine as reactive—fix the problem when it shows up. But now, with AI, we can shift to a preventative model. We’re catching diseases before they even begin.”
Nia nodded. “Machine learning in healthcare is about staying ahead of illness, not waiting for it to catch up.”
AI-Powered Surgery: Precision and Skill Combined
Next, they visited the Surgical Suite, where AI-assisted robots worked side by side with human surgeons. Here, precision was the name of the game. Machine learning algorithms helped the AI refine its skills with each surgery, becoming more accurate with every procedure.
Orion watched as robotic arms moved with absolute precision, making micro-adjustments based on real-time feedback from the patient’s body.
“This is next-level,” Orion said. “The machine is learning during the surgery, analyzing every move the surgeon makes and then adjusting its own technique. It’s like it’s getting smarter every second.”
Nia stepped closer to the hologram of the surgery in progress. “The robot is learning from past surgeries, improving its precision with each procedure. It’s not just mimicking human surgeons—it’s enhancing their skills.”
Orion smiled. “Humans and machines working together to perform surgeries that would be impossible for either one alone. It’s amazing.”
Nia added, “Exactly. The AI is learning the delicate art of surgery, combining the intuition of human surgeons with the precision of machines.”
The Human Element: AI and Compassion in Healthcare
As they returned to the central hub of MedSys, Orion and Nia stood in the middle of the AI-powered hospital, reflecting on the incredible advancements they had witnessed.
“AI is transforming healthcare,” Orion said quietly. “From diagnosing conditions to predicting illnesses and even performing surgeries. But there’s something about healthcare that machines can’t replicate—human compassion.”
Nia smiled softly, her tattoos glowing faintly. “That’s the balance, isn’t it? Machine learning is giving us the tools to improve patient outcomes in ways we never imagined. But at the end of the day, AI doesn’t comfort a worried patient or provide the emotional support that comes with a human touch.”
Orion nodded. “AI is a powerful tool in healthcare, but it’s still just a tool. The real healing comes from the connection between patients and their doctors, the empathy and understanding that no machine can ever replace.”
Nia’s eyes sparkled with hope. “The future of healthcare is about machines and humans working together. AI will handle the data, the predictions, the precision. But it’s up to us to bring the heart and soul into medicine.”
As they left MedSys, Orion and Nia knew that while machine learning was revolutionizing healthcare, the human element was—and always would be—irreplaceable.
Module 4: The Impact of AI on Job Automation
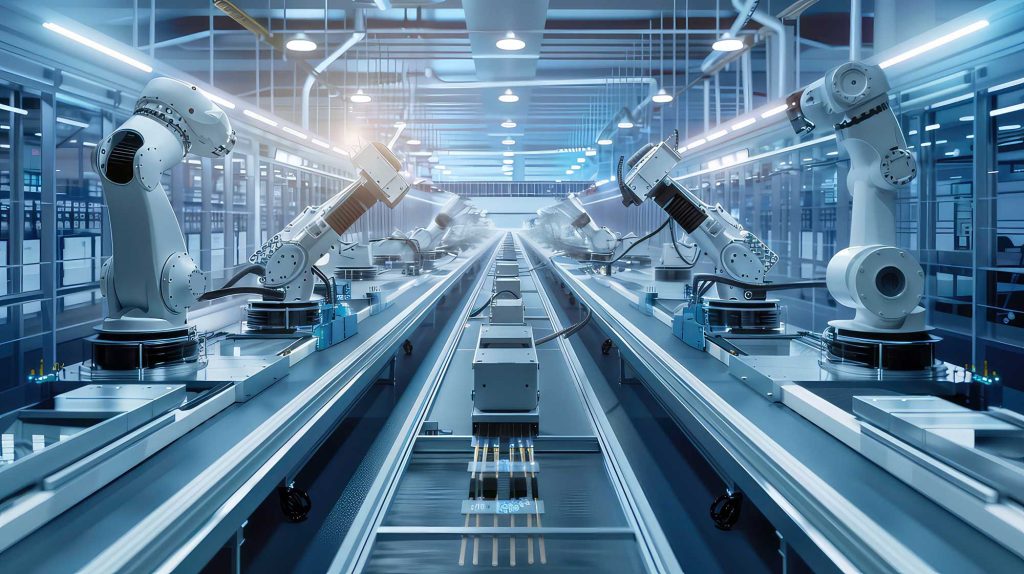
The hum of robotic arms filled the air as Orion and Nia stood in RoboWorks, a cutting-edge manufacturing facility where AI-powered robots and machines were working tirelessly. Automated systems assembled everything from vehicles to delicate electronics with mechanical precision. Conveyor belts moved at lightning speed, and humanoid robots supervised the production lines, making real-time adjustments based on data.
“Welcome to the future of work,” Nia said, her circuit-like tattoos glowing brightly as she scanned the factory floor. “Here, everything is automated, from manufacturing to logistics. AI is running the show.”
Orion gazed at the seemingly flawless systems, his holographic tablet displaying real-time data about the robots’ efficiency. “It’s impressive. But as AI continues to take over tasks traditionally handled by humans, what happens to the people who used to work these jobs?”
Nia’s expression turned thoughtful. “That’s the big question, isn’t it? Automation is changing the landscape of work, but it’s also creating a lot of uncertainty.”
Automation in Manufacturing: Efficiency at a Cost
They watched as a humanoid robot on the assembly line scanned the parts in front of it, its AI brain calculating the best way to optimize the process. With machine-like efficiency, it moved the pieces into place and welded them with precision no human could match.
Orion shook his head in awe. “This level of efficiency is incredible. The AI doesn’t need breaks, doesn’t make mistakes, and can work 24/7. It’s no wonder companies are turning to automation.”
Nia nodded. “Automation in manufacturing is a game-changer. It’s making production faster and cheaper. But we can’t ignore the downside—many of the jobs humans once held are being replaced by machines.”
She tapped on her wristband, and the holographic display shifted to show statistics of industries across the globe. “Jobs in factories, transportation, even retail—these sectors are seeing the most dramatic shifts. AI is taking over repetitive tasks, and humans are being pushed out.”
Orion frowned. “So, what’s the solution? We can’t stop progress, but we also can’t leave people behind.”
Nia’s tattoos flickered as she considered the dilemma. “The solution isn’t to stop AI from advancing. It’s to ensure we’re preparing people for the jobs of the future—roles where creativity, problem-solving, and human empathy are irreplaceable.”
AI in Retail: Automation Meets Customer Service
They walked through the automated Retail Hub, where humanoid robots greeted customers, processed payments, and even stocked shelves. Every task was handled by AI, creating a seamless shopping experience.
Orion observed as a robot assisted a customer in selecting products. “Even retail jobs are becoming automated. AI is replacing customer service reps, cashiers, and stock clerks.”
Nia sighed. “It’s not just about replacing tasks. AI can analyze customer preferences, suggest products, and even handle personalized marketing—all faster than a human ever could. For businesses, that’s invaluable.”
Orion glanced at the humanoid robots with a mixture of admiration and concern. “But what happens to the people who used to work here? What do they do now?”
Nia’s voice softened. “That’s the challenge we’re facing. Automation isn’t just about efficiency—it’s about changing the entire structure of employment. We need to think beyond traditional jobs and focus on new opportunities that AI can create.”
AI in Logistics: The Rise of Autonomous Systems
Next, they entered the Logistics Control Room, where fleets of autonomous drones and trucks handled deliveries across the city. The AI-controlled fleet monitored traffic patterns, weather conditions, and delivery routes in real time, optimizing every movement for speed and efficiency.
Nia pointed to the control panel, which was entirely operated by AI. “Look at this. AI is managing the entire logistics network. No human drivers, no dispatchers—everything is automated. It’s the future of delivery.”
Orion nodded, watching the holographic display that showed the drones delivering packages across the city. “It’s incredibly efficient. But with truck drivers and delivery workers being replaced by machines, we’re facing a massive shift in employment.”
Nia tapped her wristband, bringing up data on employment trends. “In logistics, AI is already making huge strides. But instead of seeing this as a job killer, we need to look at how AI is creating new opportunities in areas like drone management, AI system maintenance, and logistics analysis.”
Orion glanced at the data. “So, it’s not about the jobs disappearing—it’s about the jobs changing.”
Nia smiled. “Exactly. The future of work is going to be about how we adapt to these changes. AI is creating new industries, but it’s up to us to prepare people for those roles.”
The Human Factor: Skills That AI Can’t Replace
As they continued through RoboWorks, they arrived at the Innovation Lab, where AI engineers were working alongside humanoid robots to develop new technologies. Here, humans and machines were collaborating in ways that pushed the boundaries of innovation.
Orion paused, watching an engineer working with an AI assistant to develop a new prototype. “This is where humans and machines shine together. AI can handle the data and the calculations, but it’s the humans who bring creativity and intuition to the table.”
Nia’s tattoos glowed softly as she nodded. “That’s the key. AI can do a lot, but there are still things it can’t replace—like human creativity, emotional intelligence, and critical thinking. These are the skills we need to focus on as automation continues to grow.”
Orion agreed. “As more jobs become automated, the demand for skills that machines can’t replicate will increase. We need to rethink education and training to focus on developing these uniquely human abilities.”
Nia smiled. “Exactly. AI isn’t the end of work—it’s the beginning of a new era where humans can focus on the things that truly make us special.”
Rethinking the Future of Work
Back in the central hub of RoboWorks, Orion and Nia stood in front of a massive holographic display that showcased the impact of AI on industries across the globe.
Orion sighed. “AI is reshaping the workforce. But it doesn’t have to be something to fear—it can be an opportunity. If we prepare people for the new roles that AI creates, we can build a future where humans and machines work together.”
Nia’s tattoos pulsed as she considered the possibilities. “The future of work is about adaptation. We can’t stop AI from automating tasks, but we can focus on the jobs where humans excel—where creativity, empathy, and problem-solving are irreplaceable.”
Orion smiled, feeling a sense of optimism. “So, instead of fighting automation, we embrace it. We create a world where AI handles the repetitive tasks, and humans focus on what makes us uniquely human.”
As they left RoboWorks, both Orion and Nia knew that the future of job automation wasn’t about replacing humans—it was about elevating them to new heights, where their skills and creativity could truly shine.
Module 5: Reinforcement Learning—How Machines Learn from Experience
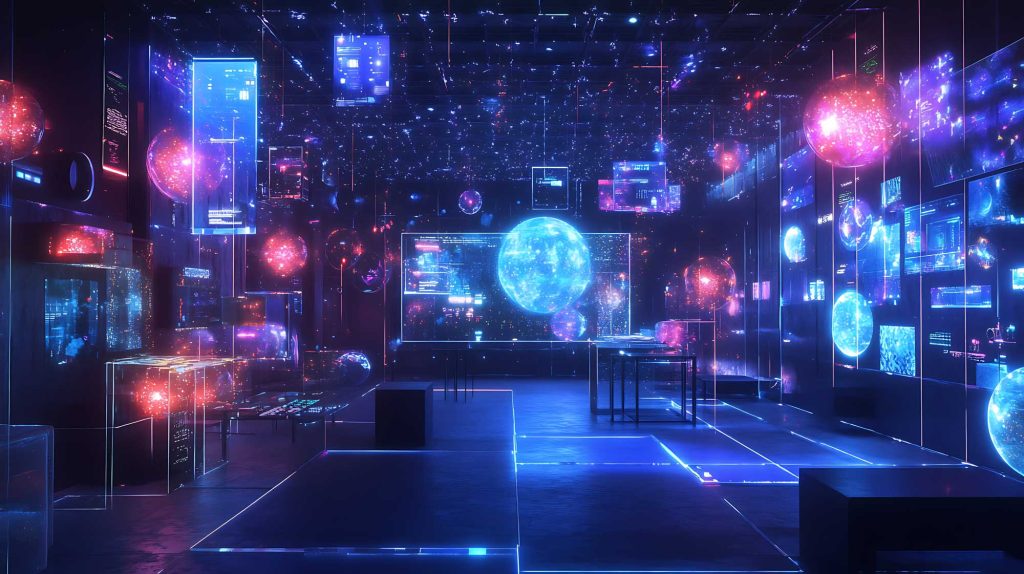
Orion and Nia stood in front of a towering holographic structure in Synapse Lab, where the latest AI development was taking place. The structure resembled a digital tree, its branches made up of nodes that glowed and shifted, representing the countless decisions being made by the AI in real-time. This was the heart of reinforcement learning, the branch of AI that allowed machines to learn from their actions and continuously improve based on feedback.
“This is it, Orion,” Nia said, her circuit-like tattoos flickering with excitement. “Reinforcement learning in action. The AI learns from its environment, makes decisions, and improves based on the rewards or consequences it encounters.”
Orion adjusted his holographic tablet, bringing up the live data feed. “So, this is how machines truly learn through experience? Like a trial-and-error process, but they get smarter each time?”
Nia nodded. “Exactly. They make decisions, see the outcomes, and adjust their behavior to maximize the reward. It’s the closest we’ve come to mimicking how humans and animals learn through experience.”
Learning Through Trial and Error: A Game of Chess
Nia pulled up a holographic simulation of a chessboard. “Let’s start with something simple. The AI here has been learning to play chess using reinforcement learning. Every time it makes a move, it either wins or loses points based on the outcome.”
Orion watched as the AI played against a virtual opponent, making quick moves across the chessboard. “So, every move it makes teaches it something. The more it plays, the better it gets, right?”
Nia smiled. “Exactly. At first, the AI made random moves, but over time, it learned which strategies led to better outcomes. Now, it’s playing at a level far beyond human ability because it’s continuously learning from every game.”
The AI made a brilliant checkmate in just a few moves, having optimized its strategy through countless games. Orion raised an eyebrow. “It’s like watching a grandmaster that never tires, never forgets, and never stops learning.”
Reinforcement Learning in Robotics: Mastering Complex Tasks
Next, they moved to the robotics section of the lab, where a humanoid robot was using reinforcement learning to assemble a complex piece of machinery. The robot had no prior instructions—it was learning entirely through trial and error.
“Watch this,” Nia said, pointing at the robot as it manipulated different parts. “It’s learning how to assemble this machinery just by trying different approaches. Every time it makes a mistake, it learns from it. And when it succeeds, it reinforces that behavior.”
Orion observed as the robot initially fumbled with the parts, struggling to fit them together. But after several attempts, it adjusted its grip, learned the correct sequence, and completed the assembly with precision.
“It’s fascinating,” Orion said. “The robot is teaching itself. It wasn’t programmed to know how to do this—it figured it out through experience.”
Nia nodded, her tattoos glowing softly. “That’s the beauty of reinforcement learning. The machine isn’t just following instructions. It’s learning how to adapt, how to improve, and how to perform complex tasks that it wasn’t explicitly programmed for.”
Reinforcement Learning in the Real World: Autonomous Systems
They walked over to a larger holographic display that showed the layout of Neon Valley, their futuristic city. Autonomous vehicles zipped through the streets, drones made deliveries, and energy systems adjusted based on real-time data. All of it was managed by AI systems that used reinforcement learning to optimize their performance.
Orion pulled up the live data of an autonomous vehicle navigating the city. “So, these cars are using reinforcement learning too? They’re learning how to drive based on feedback from the environment?”
Nia smiled. “Exactly. The vehicles started by learning basic driving patterns, but over time, they’ve encountered every kind of scenario—traffic jams, accidents, bad weather. Each time they face a challenge, they learn from it, and the system improves.”
Orion watched as the vehicle made an unexpected detour to avoid construction, seamlessly adapting to the change in its environment. “It’s almost like they’re developing instincts, like a driver who knows the shortcuts in their hometown.”
Nia’s eyes twinkled. “That’s the goal. The more the system learns from its environment, the better it gets at navigating the complexities of the real world. And because it’s learning from experience, every vehicle in the system benefits from what all the others have learned.”
Challenges of Reinforcement Learning: The Balance Between Exploration and Exploitation
As they continued, Nia pulled up a complex graph showing the balance between two key concepts in reinforcement learning: exploration and exploitation.
“Here’s the challenge,” Nia explained. “The AI has to strike a balance between exploring new strategies—trying new things—and exploiting what it already knows—sticking to what works. If it explores too much, it wastes time trying things that don’t work. But if it exploits too much, it might miss out on better solutions.”
Orion studied the graph. “So, it’s constantly making decisions about whether to take risks and explore or stick with what it knows. Just like humans do.”
Nia nodded. “Exactly. And this balance is critical. If the AI explores too little, it stagnates. If it explores too much, it becomes inefficient. The key to success in reinforcement learning is finding that sweet spot.”
She tapped her wristband, and the graph shifted to show how the AI had fine-tuned its strategy over time, becoming more efficient as it learned to balance exploration and exploitation.
The Future of Reinforcement Learning: Beyond Machines
As they left the robotics section and returned to the heart of Synapse Lab, Orion and Nia reflected on the future of reinforcement learning. It wasn’t just about machines learning from experience—it was about the potential to apply this kind of learning across industries.
Orion looked thoughtful as he glanced at the AI systems running throughout the lab. “If machines can learn from experience and continuously improve, what does that mean for industries beyond robotics? Could reinforcement learning be used in education, healthcare, even environmental sustainability?”
Nia’s tattoos glowed brighter as she nodded. “Absolutely. Imagine a world where AI systems in hospitals are constantly improving their treatments based on patient outcomes, or where education systems adapt to each student’s learning style in real time. Reinforcement learning could revolutionize every field it touches.”
Orion smiled, feeling the weight of the possibilities. “We’re not just building smarter machines. We’re building systems that can adapt, grow, and learn—just like we do.”
Nia grinned. “And that’s the future of AI. Reinforcement learning is taking us beyond programming into a world where machines learn from the world around them, making decisions that continuously get better.”
As they stood in the futuristic lab, surrounded by the hum of innovation, both Orion and Nia knew that they were on the cusp of a new era—one where machines didn’t just execute tasks but learned, evolved, and reshaped the world alongside humanity.